Forecasting online products
- Marie-Antoinette Mbaye
- 24 oct. 2023
- 3 min de lecture
Since the beginning of the online area, companies have been facing the same challenges concerning the forecasting to which specific contextual problems are added. Thus, they encounter difficulties for predicting customer demand, especially when they are a click away from their next competitors. Due to these specificities, online business appears to be highly competitive and requires its own process.
Already aware of this question in 2001, Golicic et al.[1], has determined that there are internal and external factors affecting the forecasting effectiveness. If online business is intense, connected, and dynamic, it then requires business decisions in real-time due to the rapidity of the actions and the fast product update.
Moreover, e-commerce business breaks the geographical barriers, it also touches customers from different profiles than physical retail. In that case, the role of the supply chain is to deliver a solution that can fit the final customer demand.
For researchers, the “natural” way to forecast is by using quantitative methods. However, time series and regression require historical data. We should underline that e-commerce business has a lot of newness products and a high level of product rotation. In that case, it might be difficult to forecast with quantitative methods.
Another option is then possible: the use of qualitative methods when no data are available and high uncertainty are present. Qualitative methods rest on internal and external “experts”. Internal experts can be the sales manager, while external experts can be customers’ feedbacks. Indeed, gathering customers' expectations or behaviors can be a good way to assess the demand. By using surveys or CRM tools, companies can have more information about their customers’ profiles. This is the real strength of the internet business compared to physical sales. Using qualitative data from customers can then lead to the projection of future products and services needed.
Other than the method used, Golicic et al. (2001), focused on the parameters that can influence the demand. Indeed, recording all the branding and communication events like public relationship, promotion, media diffusion, or advertisement, allows to track the leverages on sales and how they impact the demand. If companies have a better knowledge of the impact of branding and communication strategies, they can anticipate sales lift and match capacities.
For the forecasting method, even if the quantitative method is applicable in most cases, adding the qualitative aspect with the customers’ perspectives can increase the forecast accuracy. Indeed, CRM tools on online retail like SalesForce can help to analyze the customer route on the site and give feedback on the lost sales due to shortages, or most consulted items with low sales. Within this method, technological solutions are excellent tools to collect, process, and analyze the customer data.
In another hand, companies should take a look on the impact of branding, communication, and promotion events on their sales. However, my research in cosmetics and textile have shown that not all the companies are assessing the impact of product exposure or promotion on their sales. Even if planners still have the possibility to express lift periods (meaning an increase of sales outside of the seasonality), this solution is not enough. Companies should keep track and record the impact of each kind of branding action or animation per reference.
This database can be alimented by the supply chain for the sales and the marketing communication for the qualification of the “event”. For example, the cosmetic field is well known for its paid partnerships with influencers on social media. Tracking the sales made after a post on Instagram can help the marketing department to decide if the partnership was effective while giving an idea of the impact on retail and online demand.
[1] Golicic S.L., et al., (2001), ‘Bringing Order out of Chaos: Forecasting E-Commerce’, Journal of Business Forecasting Methods & Systems, 20(1), p.11-17.
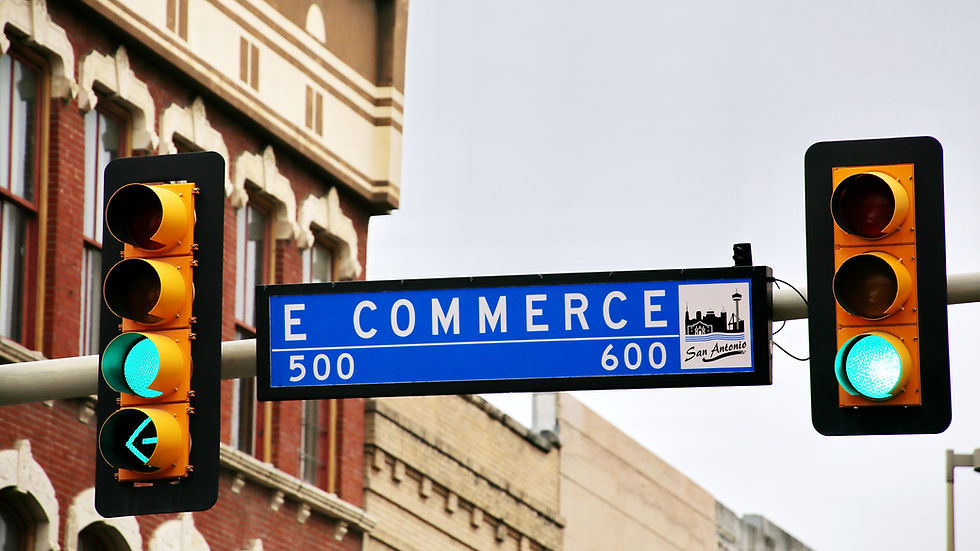
Comments